Artificial Intelligence (AI) is transforming industries across the globe, from healthcare and finance to gaming and autonomous vehicles. As AI adoption soars, NVIDIA, a leader in GPU technology, has been instrumental in developing tools and frameworks that make AI development accessible and efficient. Among their latest offerings, the NVIDIA TAO Toolkit stands out as a revolutionary platform, reshaping the way developers and researchers create customized AI models. In this extensive blog post, we will explore the NVIDIA TAO Toolkit in-depth, covering its features, applications, benefits, challenges, and impact on the AI landscape.
Understanding the NVIDIA TAO Toolkit
The NVIDIA TAO Toolkit, an acronym for “Train, Adapt, and Optimize,” is a comprehensive suite of tools and libraries developed to simplify and accelerate AI model development. It provides a unified platform that spans the entire AI development pipeline, offering capabilities for training models from scratch, adapting pre-trained models to specific tasks, and optimizing models for deployment across various hardware platforms.
TAO comprises several interconnected components, each tailored to different stages of the AI development process:
- TAO Training : This component enables users to train AI models from the ground up, tailored to their specific requirements. TAO Training supports a variety of neural network architectures and offers pre-configured training recipes for tasks such as object detection, image segmentation, and more.
- TAO Toolkit : The TAO Toolkit streamlines the process of fine-tuning pre-trained models for domain-specific tasks. It includes a rich repository of pre-trained models and facilitates transfer learning, allowing developers to adapt these models to new use cases with relative ease.
- TAO Optimizer : Once a model is trained and adapted, the TAO Optimizer comes into play, optimizing the model's performance for deployment. This optimization includes techniques like model quantization and pruning, reducing the model's size and enhancing its efficiency.
Transfer Learning Made Easy
TAO simplifies the intricate process of transfer learning by providing a repository of pre-trained models. These models have been trained on vast datasets, enabling developers to fine-tune them for specific tasks using relatively small amounts of task-specific data. This significantly reduces the time and resources needed for training custom AI models.
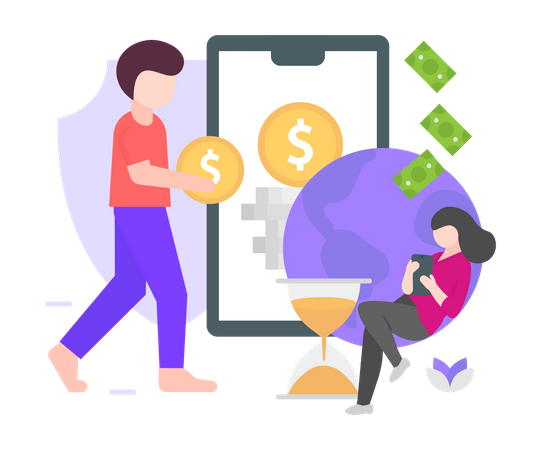
Performance Optimization
Efficient deployment of AI models is crucial for real-world applications. TAO’s optimization features play a pivotal role in achieving this goal. By reducing the model’s size and computational requirements, AI applications can run seamlessly on edge devices and embedded systems, expanding the horizons of AI deployment.
Customization and Adaptation
The TAO Toolkit offers a broad spectrum of model architectures, empowering users to select the one that aligns best with their project requirements. Moreover, it supports the adaptation of models across diverse domains, making it an ideal choice for applications in fields such as healthcare, robotics, and automotive.
Efficient Data Augmentation
TAO includes data augmentation techniques that enhance model robustness by generating variations of the training data. This becomes especially crucial when working with limited training datasets, as it mitigates overfitting and improves model generalization.
Comprehensive Hardware Support
NVIDIA has designed TAO to be compatible with a wide range of hardware platforms, from powerful GPUs to energy-efficient NVIDIA Jetson devices. This flexibility ensures that AI models built with TAO can be deployed across diverse devices, catering to a multitude of use cases.
Extensive Documentation and Community Support
NVIDIA’s commitment to user-friendliness is reflected in the extensive documentation and resources provided for TAO. Additionally, there exists a vibrant community of AI enthusiasts, developers, and researchers actively sharing their experiences and solutions. This collaborative ecosystem further facilitates the adoption and growth of the TAO Toolkit.
Applications of the NVIDIA TAO Toolkit
The versatility of the NVIDIA TAO Toolkit has led to its adoption across various industries, significantly accelerating the development of AI solutions. Let’s explore some notable applications:
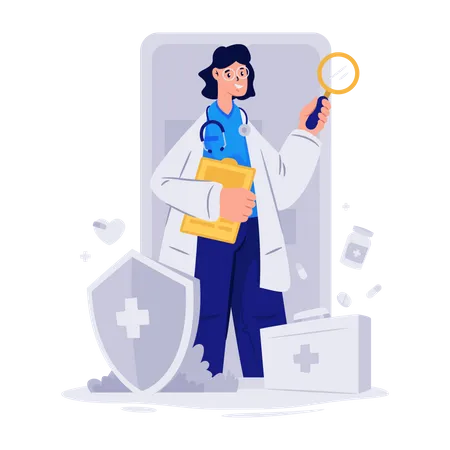
Healthcare
TAO is instrumental in adapting pre-trained models for medical image analysis. Tasks like anomaly detection in X-rays, segmentation of MRI scans, and disease classification benefit greatly from TAO’s capabilities. Its adaptability allows researchers to fine-tune models for specific diseases or medical conditions, making it an invaluable asset in the healthcare sector.
Retail
Retailers are leveraging the TAO Toolkit to create custom AI models for inventory management, demand forecasting, and customer behavior analysis. These applications improve inventory turnover rates and enhance the overall shopping experience, optimizing retail operations.
Autonomous Vehicles
The development of self-driving cars relies heavily on AI for perception and decision-making. TAO simplifies the training of models for tasks like object detection, lane detection, and pedestrian tracking, contributing to the advancement of autonomous vehicle technology.
Manufacturing
In the manufacturing sector, TAO is applied to quality control tasks, defect detection in production lines, and predictive maintenance. These applications reduce downtime, minimize defects, and improve production efficiency, resulting in significant cost savings.
Natural Language Processing (NLP)
TAO is equally adept at fine-tuning pre-trained language models. This capability is invaluable for applications such as sentiment analysis, chatbots, document summarization, and more, enhancing human-computer interaction in various contexts.
Robotics
AI-powered robots benefit from TAO’s adaptability. They can perform an array of tasks, ranging from object manipulation to navigation in dynamic and unstructured environments. This flexibility opens doors to a multitude of robotic applications.
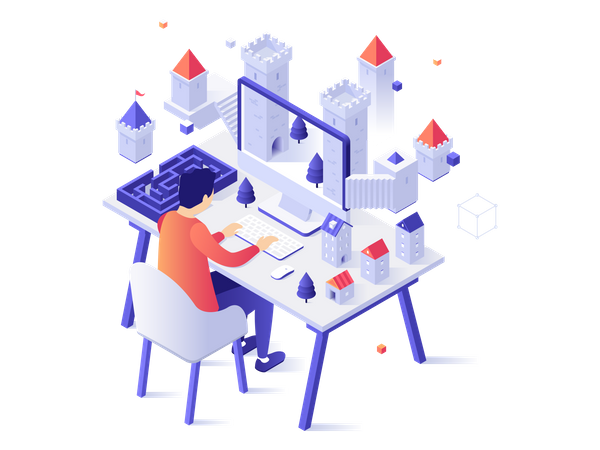
Benefits of Using the NVIDIA TAO Toolkit
The adoption of the NVIDIA TAO Toolkit brings forth a multitude of advantages for organizations and developers immersed in AI development:
Accelerated Development
TAO significantly reduces the time and effort required for AI model development. This acceleration empowers organizations to remain competitive in rapidly evolving markets, allowing them to deliver AI-powered solutions to their customers swiftly.
Enhanced Model Performance
Through the fine-tuning and optimization capabilities provided by TAO, developers can achieve higher accuracy and efficiency in their AI applications. This is pivotal for ensuring that AI models meet the rigorous demands of real-world scenarios.
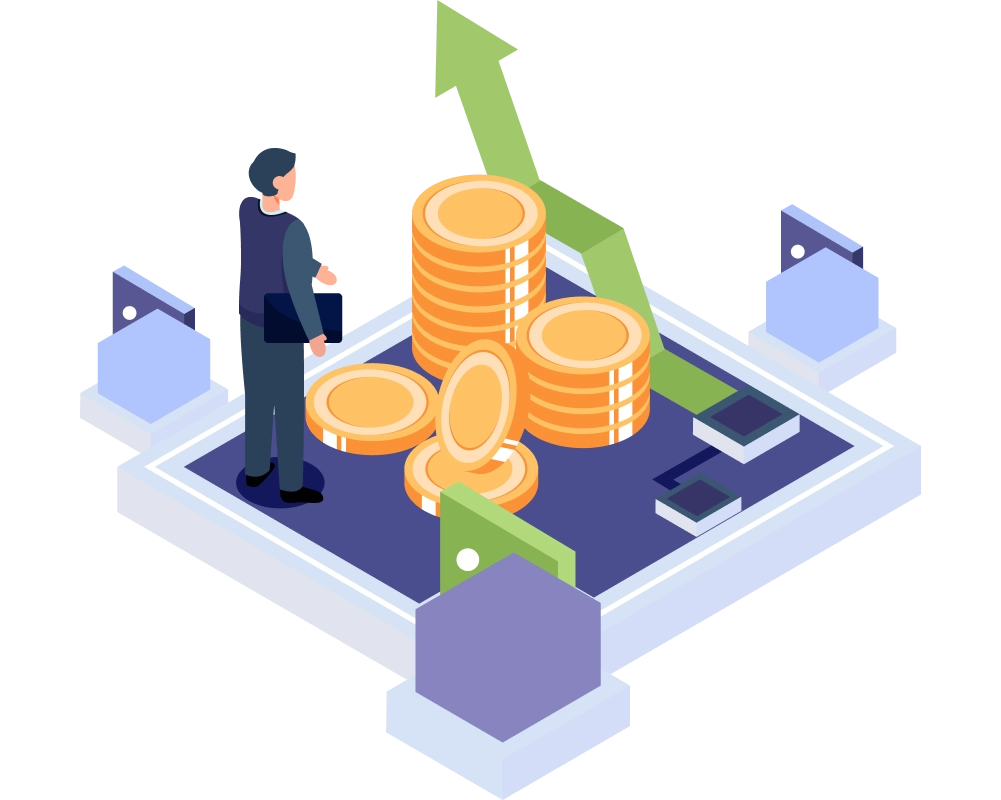
Cost-Effectiveness
By enabling transfer learning and model adaptation, TAO can significantly reduce the costs associated with collecting and annotating large datasets. This cost-effectiveness enhances the feasibility of AI projects, especially for smaller organizations and startups.
Resource Efficiency
The model optimization features within the TAO Toolkit enable the deployment of AI models on resource-constrained devices.
Resource Efficiency
This capability broadens the scope of AI deployment to edge devices and embedded systems, creating new opportunities and use cases.
Challenges and Considerations
While the NVIDIA TAO Toolkit offers compelling advantages, it’s essential to consider the challenges and considerations that may arise during its utilization:
Data Privacy
AI models trained with TAO may still require access to sensitive data for customization. Ensuring data privacy and compliance with regulations like GDPR is paramount to avoid legal and ethical issues.
Model Interpretability
As models become more complex, understanding their decision-making processes can be challenging. Interpretability remains an active area of research, and developers must consider how to make AI models more transparent and accountable.
Resource Constraints
Deploying AI models on edge devices may present challenges related to computational resources and power consumption. Careful resource management and optimization are required to ensure optimal performance.
Continuous Learning
Deploying AI models on edge devices may present challenges related to computational resources and power consumption. Careful resource management and optimization are required to ensure optimal performance.
Conclusion
The NVIDIA TAO Toolkit emerges as a pivotal advancement in AI model development, democratizing the creation of custom AI solutions. By simplifying the intricacies of training, adaptation, and optimization, TAO empowers developers, researchers, and organizations to harness the power of AI with unprecedented ease and efficiency. Its applications span across diverse industries, ranging from healthcare and autonomous vehicles to retail and manufacturing. As AI continues to reshape the world, TAO stands as a valuable tool for those seeking to push the boundaries of what’s possible. With ongoing development and the support of a vibrant NVIDIA community, TAO promises to play a pivotal role in the future of artificial intelligence, ushering in an era of unprecedented AI innovation and deployment.