Prior, in-depth review of plant conditions, careful financial and material preparation, and adequate training of maintenance personnel will greatly minimize implementation time.
Predictive maintenance (PdM) is a constructive, data-driven maintenance strategy that monitors real-time equipment working conditions in order to anticipate potential equipment breakdown and create reliable maintenance schedules. As a technique for driving manufacturing productivity, it is gaining favor over conventional approaches such as six sigma and lean management. PdM systems are capable of cutting operating costs by 12%, increasing machine uptime by 9%, expanding equipment lifespan by more than 20%, and lowering health and environmental, and quality risks by 14%.
Here are few considerations for plant managers and technicians when transitioning to predictive maintenance.
1. Infrastructure requirements
Sensors are used in predictive maintenance to gather real-time data from plant machinery. The sensors are capable of tracking and identifying even the smallest variations in the activity of the equipment. Temperature, strain, acceleration, rotational speeds, and other parameters are collected by condition-based control utilities. Adjustments should also be made to avoid minor flaws from escalating into significant faults that could cripple plant processes.
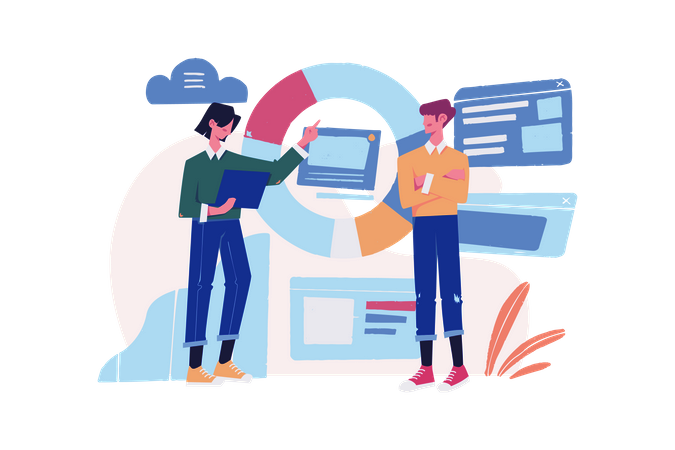
Converting sensor data into warning signals and usable information necessitates a strong infrastructure comprised of both software and hardware systems. These systems should be able to measure and process data in real-time, as well as manage data from a wide range of sensors.
Until implementing a PdM system, define plant requirements and pick suitable sensors and system equipment capable of collecting, converting, and managing sensitive machine data.
2. Data management
Sensors installed on sensitive plant machinery will gather massive quantities of data at any given time. In its raw form, this data can be daunting. When transitioning to a PdM system, it is crucial that the system be capable of filtering and mapping critical data from all sensors.
The PdM algorithms and predictive models must be able to forecast errors and failure modes based on the data that has been studied. The data-management framework is intended to have a user-friendly interface in which complicated data is presented in basic and readable formats (graphs and pictorials), in which constructive maintenance can be performed.
PdM applications should be able to consistently gather data on sensitive assets without interfering with plant operations or the operational capability of the data-management platform.
3. User adoption and training
The transition to predictive maintenance will expose the maintenance staff to new systems, software, and procedures. A PdM framework would inevitably interrupt current maintenance processes, so it is critical that workers consider the additional software requirements and modifications that will result from its introduction.
The smooth transition to a PdM maintenance strategy would be dependent on user acceptance and maintenance teams’ good comprehension of processes. Furthermore, developing data models that can be used to forecast equipment breakdowns is a difficult process that often necessitates the hiring of additional personnel, such as data scientists and reliability engineers.
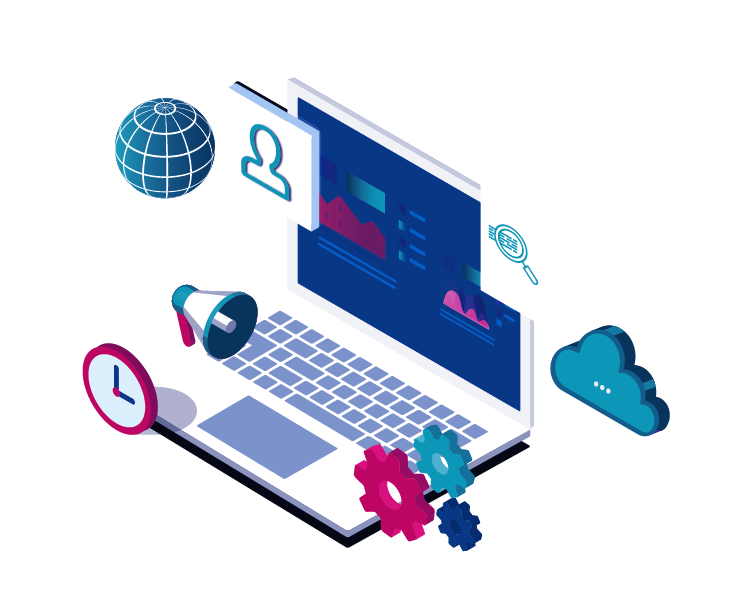
4. Ease of monitoring and alert generation
It is important that you use an easy-to-monitor framework before implementing predictive maintenance. Apart from breaking down complex data sets into understandable analytics, it is often critical to identify errors and generate relevant warnings. Alerts are used as input mechanisms in PdM systems to ensure efficient coordination with devices and repair teams.
A PdM framework must be capable of identifying and avoiding false alarms, as well as producing standardized alerts based on relevant data points. Generic warnings are preferred to accurate alerts that pinpoint the origin or root cause of errors. Companies may create a robust failure mode and root cause analysis model by creating a database with periodic warnings and error sources.
5. Dealing with security issues
Predictive management connects industrial infrastructure to a variety of cloud computing services and is made possible by IoT networking. These systems are needed for better real-time data analysis and storing of equipment performance metrics.
Once plant infrastructure is linked to these systems, maintenance goes beyond physical defense, incorporating cybersecurity techniques into industrial asset management.
The security of IoT and cloud infrastructure technologies used in PdM systems should be prioritized. Several threats and security breaches on vital infrastructure installations have occurred around the globe. There have been allegations of serious cyberattacks mostly targeting Industrial IoT technologies. A PdM system must provide comprehensive security capabilities to protect against potential internal and external threats.
6. Starting with a pilot project
It is important to develop a strategic action strategy before implementing a predictive maintenance model. Implementation preparation defines essential plant infrastructure and points out potential implementation bottlenecks that could occur as a result of implementing a predictive maintenance scheme.
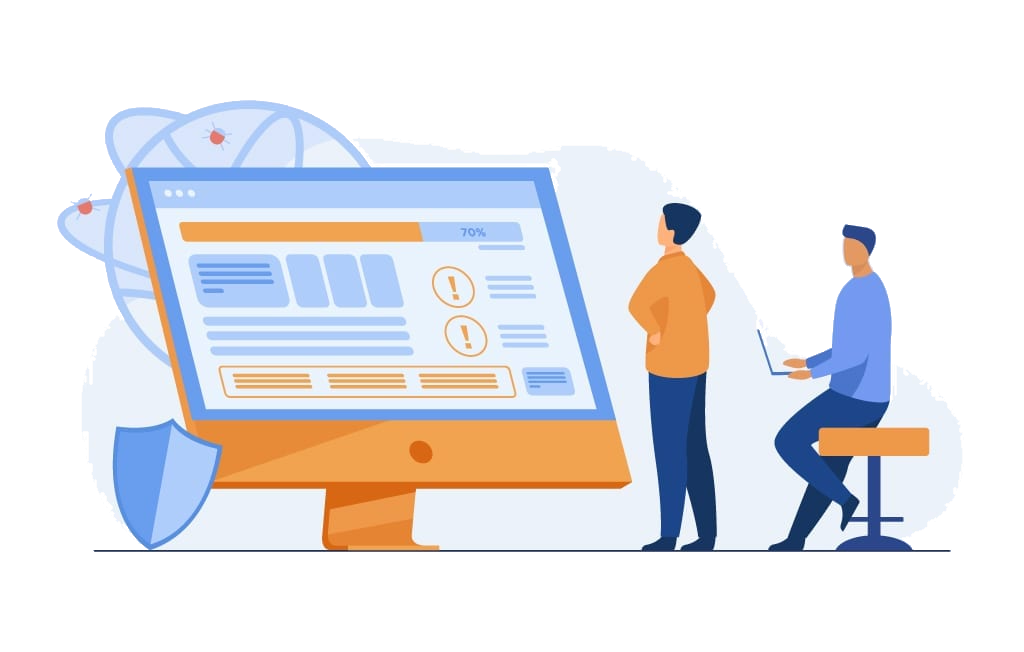
When a pilot project is applied ahead of time, transitioning to a PdM framework can take less time. The pilot project will be used to train employees, validate system security, and improve predictive algorithms.
Summing Up
Many industries are transitioning from reactive to predictive maintenance techniques. This has been accelerated by the advancement of IoT technologies, IoT– enabled CMMS solutions, and the fact that deployment costs are continuously decreasing.
It takes time to completely enforce the PdM approach. Implementation challenges can be greatly minimized by doing a pre, in-depth review of plant needs, appropriate financial and material preparation, and adequate training of maintenance staff.